Researchers Match Deep Learning Methods to Microscopy Applications
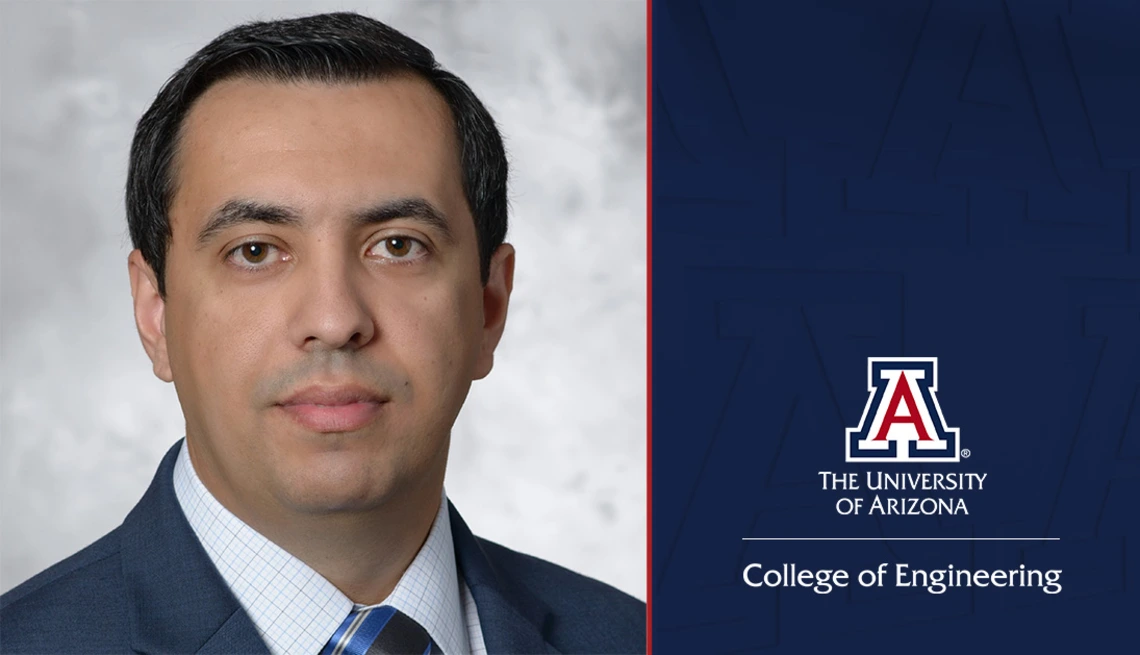
Deep learning is a type of artificial intelligence algorithm that uncovers complex data patterns which are otherwise challenging and time-consuming to identify. Its popularity has exploded in many fields, including microscopy image analysis.
In a review paper published yesterday, July 26 in Progress in Materials Science, University of Arizona and University of Illinois researchers have developed recommendations for which deep learning methods are optimal for the most critical tasks in microscopy image processing. After combing through hundreds of papers on the topic, they grouped deep learning tasks for materials science and engineering into four categories: synthetic data generation, materials structure identification, 3D visual reconstruction based on a 2D image, and physical properties evaluation.
The UA authors are Vitaliy Yurkiv, an assistant professor, and Farzad Mashayek, professor and head of the Aerospace and Mechanical Engineering Department. Their collaborators at the University of Illinois at Chicago is Reza Shahabazian-Yassar, professor of mechanical and industrial engineering, and Yurkiv’s former Ph.D. student Marco Ragone, who recently graduated with his Ph.D. and now works at CCC Intelligent Solutions.
Over the past few years, Yurkiv and his team developed a deep learning method that allowed researchers to reconstruct a 3D structure based on a 2D microscopy image. Prior to this development, it could take a researcher a full day to analyze and process one of these images.
“Now with machine learning, we can do it in a couple of seconds,” Yurkiv said. “Or, when an experiment recorded 1,000 images or 10,000 images, a researcher would need years to analyze them. But now, with our developed method, we can do it in one hour or less with modern graphics processing units, or GPUs.”
In this literature review, the team analyzed many different methods, including their own, and identified which combinations of methods are best suited to the four categories of applications they identified. They also suggested improvements for every approach. Ultimately, all are aimed at calculating structure-property relations, or determining how molecular-level structure predicts the quality and functionality of a material. This could help researchers determine how to engineer materials for applications such as rechargeable batteries, fuel cells and power electronics.
“It could be applied everywhere where we want to know the structure of materials,” Dr. Yurkiv said. “There is no limitation, as long as we have 2D microscopy images. If we have a good-quality image from electron microscopy, we can easily reconstruct the 3D image of anything.”
Progress in Materials Science has a five-year average impact factor of 42.9, a metric that is based on how often the papers in a journal are cited. This is the sixth highest out of 341 journals categorized under “materials science, multidisciplinary” by Elsevier.